To solve this we can use Dynamic Programming to gradually build upon previous solutions for t-1 transactions. The simplest to understand solution uses O(n²k) time and O(nk) space. We can optimise the time to O(nk) and space to O(n). The general idea of the algorithm is to step through the days of our current transaction row and see if we can add a transaction at any point of the previous row to increase the profit. See the whiteboard below to see the progression through the solutions to optimise time and space.
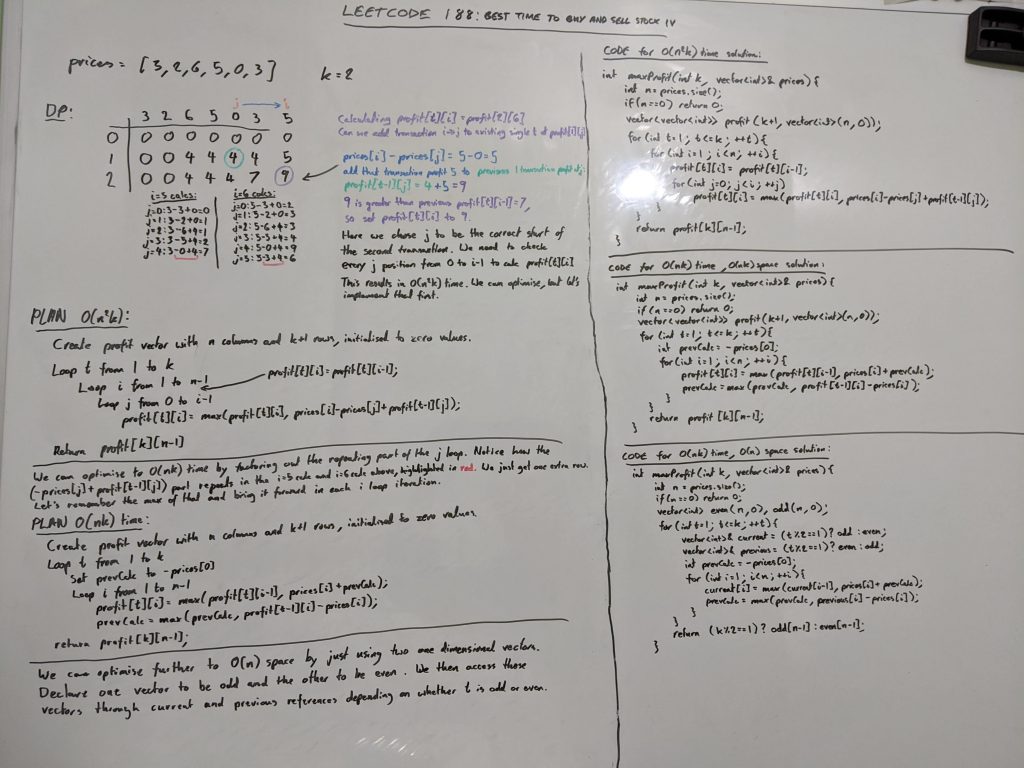
Inspired by the following great articles/videos:
https://www.geeksforgeeks.org/maximum-profit-by-buying-and-selling-a-share-at-most-k-times/
https://www.youtube.com/watch?v=Pw6lrYANjz4 (algoexpert video)